데모¶
라이브러리 import 및 설정¶
%reload_ext autoreload
%autoreload 2
%matplotlib inline
import gc
from matplotlib import rcParams, pyplot as plt
import numpy as np
import os
import pandas as pd
from pathlib import Path
import re
from sklearn.metrics import accuracy_score, log_loss
from sklearn.model_selection import StratifiedKFold
import tensorflow as tf
from tensorflow import keras
from tensorflow.keras import Sequential, layers
from tensorflow.keras.backend import clear_session
from tensorflow.keras.layers import Dense, Embedding, LSTM, GlobalMaxPooling1D, Conv1D, Dropout, Bidirectional
from tensorflow.keras.preprocessing.sequence import pad_sequences
from tensorflow.keras.preprocessing.text import Tokenizer
from tensorflow.keras.callbacks import EarlyStopping, ReduceLROnPlateau
from tensorflow.keras.utils import plot_model, to_categorical
from tensorflow.keras.optimizers import Adam
import warnings
warnings.filterwarnings(action='ignore')
gpus = tf.config.experimental.list_physical_devices('GPU')
if gpus:
# Restrict TensorFlow to only use the first GPU
try:
tf.config.experimental.set_visible_devices(gpus[0], 'GPU')
logical_gpus = tf.config.experimental.list_logical_devices('GPU')
print(len(gpus), "Physical GPUs,", len(logical_gpus), "Logical GPU")
except RuntimeError as e:
# Visible devices must be set before GPUs have been initialized
print(e)
else:
print('No GPU detected')
1 Physical GPUs, 1 Logical GPU
rcParams['figure.figsize'] = (16, 8)
plt.style.use('fivethirtyeight')
pd.set_option('max_columns', 100)
pd.set_option("display.precision", 4)
warnings.simplefilter('ignore')
학습데이터 로드¶
data_dir = Path('../data/dacon-author-classification')
feature_dir = Path('../build/feature')
val_dir = Path('../build/val')
tst_dir = Path('../build/tst')
sub_dir = Path('../build/sub')
dirs = [feature_dir, val_dir, tst_dir, sub_dir]
for d in dirs:
os.makedirs(d, exist_ok=True)
trn_file = data_dir / 'train.csv'
tst_file = data_dir / 'test_x.csv'
sample_file = data_dir / 'sample_submission.csv'
target_col = 'author'
n_fold = 5
n_class = 5
seed = 42
algo_name = 'mta'
feature_name = 'emb'
model_name = f'{algo_name}_{feature_name}'
feature_file = feature_dir / f'{feature_name}.csv'
p_val_file = val_dir / f'{model_name}.val.csv'
p_tst_file = tst_dir / f'{model_name}.tst.csv'
sub_file = sub_dir / f'{model_name}.csv'
train = pd.read_csv(trn_file, index_col=0)
train.head()
text | author | |
---|---|---|
index | ||
0 | He was almost choking. There was so much, so m... | 3 |
1 | “Your sister asked for it, I suppose?” | 2 |
2 | She was engaged one day as she walked, in per... | 1 |
3 | The captain was in the porch, keeping himself ... | 4 |
4 | “Have mercy, gentlemen!” odin flung up his han... | 3 |
test = pd.read_csv(tst_file, index_col=0)
test.head()
text | |
---|---|
index | |
0 | “Not at all. I think she is one of the most ch... |
1 | "No," replied he, with sudden consciousness, "... |
2 | As the lady had stated her intention of scream... |
3 | “And then suddenly in the silence I heard a so... |
4 | His conviction remained unchanged. So far as I... |
Preprocessing¶
MultiHeadSelfAttention
and TransformerBlock
from the Text classification with Transformer tutorial at the Keras website.
class MultiHeadSelfAttention(layers.Layer):
def __init__(self, embed_dim, num_heads=8):
super(MultiHeadSelfAttention, self).__init__()
self.embed_dim = embed_dim
self.num_heads = num_heads
if embed_dim % num_heads != 0:
raise ValueError(
f"embedding dimension = {embed_dim} should be divisible by number of heads = {num_heads}"
)
self.projection_dim = embed_dim // num_heads
self.query_dense = layers.Dense(embed_dim)
self.key_dense = layers.Dense(embed_dim)
self.value_dense = layers.Dense(embed_dim)
self.combine_heads = layers.Dense(embed_dim)
def attention(self, query, key, value):
score = tf.matmul(query, key, transpose_b=True)
dim_key = tf.cast(tf.shape(key)[-1], tf.float32)
scaled_score = score / tf.math.sqrt(dim_key)
weights = tf.nn.softmax(scaled_score, axis=-1)
output = tf.matmul(weights, value)
return output, weights
def separate_heads(self, x, batch_size):
x = tf.reshape(x, (batch_size, -1, self.num_heads, self.projection_dim))
return tf.transpose(x, perm=[0, 2, 1, 3])
def call(self, inputs):
# x.shape = [batch_size, seq_len, embedding_dim]
batch_size = tf.shape(inputs)[0]
query = self.query_dense(inputs) # (batch_size, seq_len, embed_dim)
key = self.key_dense(inputs) # (batch_size, seq_len, embed_dim)
value = self.value_dense(inputs) # (batch_size, seq_len, embed_dim)
query = self.separate_heads(
query, batch_size
) # (batch_size, num_heads, seq_len, projection_dim)
key = self.separate_heads(
key, batch_size
) # (batch_size, num_heads, seq_len, projection_dim)
value = self.separate_heads(
value, batch_size
) # (batch_size, num_heads, seq_len, projection_dim)
attention, weights = self.attention(query, key, value)
attention = tf.transpose(
attention, perm=[0, 2, 1, 3]
) # (batch_size, seq_len, num_heads, projection_dim)
concat_attention = tf.reshape(
attention, (batch_size, -1, self.embed_dim)
) # (batch_size, seq_len, embed_dim)
output = self.combine_heads(
concat_attention
) # (batch_size, seq_len, embed_dim)
return output
class TransformerBlock(layers.Layer):
def __init__(self, embed_dim, num_heads, ff_dim, rate=0.1):
super(TransformerBlock, self).__init__()
self.att = MultiHeadSelfAttention(embed_dim, num_heads)
self.ffn = keras.Sequential(
[layers.Dense(ff_dim, activation="relu"), layers.Dense(embed_dim),]
)
self.layernorm1 = layers.LayerNormalization(epsilon=1e-6)
self.layernorm2 = layers.LayerNormalization(epsilon=1e-6)
self.dropout1 = layers.Dropout(rate)
self.dropout2 = layers.Dropout(rate)
def call(self, inputs, training):
attn_output = self.att(inputs)
attn_output = self.dropout1(attn_output, training=training)
out1 = self.layernorm1(inputs + attn_output)
ffn_output = self.ffn(out1)
ffn_output = self.dropout2(ffn_output, training=training)
return self.layernorm2(out1 + ffn_output)
class TokenAndPositionEmbedding(layers.Layer):
def __init__(self, maxlen, vocab_size, embed_dim):
super(TokenAndPositionEmbedding, self).__init__()
self.token_emb = layers.Embedding(input_dim=vocab_size, output_dim=embed_dim)
self.pos_emb = layers.Embedding(input_dim=maxlen, output_dim=embed_dim)
def call(self, x):
maxlen = tf.shape(x)[-1]
positions = tf.range(start=0, limit=maxlen, delta=1)
positions = self.pos_emb(positions)
x = self.token_emb(x)
return x + positions
X_train = train['text'].values
X_test = test['text'].values
y = train['author'].values
print(X_train.shape, X_test.shape, y.shape)
(54879,) (19617,) (54879,)
def alpha_num(text):
return re.sub(r'[^A-Za-z0-9 ]', '', text)
def remove_stopwords(text):
final_text = []
for i in text.split():
if i.strip().lower() not in stopwords:
final_text.append(i.strip())
return " ".join(final_text)
stopwords = [ "a", "about", "above", "after", "again", "against", "all", "am", "an", "and", "any", "are", "as",
"at", "be", "because", "been", "before", "being", "below", "between", "both", "but", "by", "could",
"did", "do", "does", "doing", "down", "during", "each", "few", "for", "from", "further", "had", "has",
"have", "having", "he", "he'd", "he'll", "he's", "her", "here", "here's", "hers", "herself", "him", "himself",
"his", "how", "how's", "i", "i'd", "i'll", "i'm", "i've", "if", "in", "into", "is", "it", "it's", "its", "itself",
"let's", "me", "more", "most", "my", "myself", "nor", "of", "on", "once", "only", "or", "other", "ought", "our", "ours",
"ourselves", "out", "over", "own", "same", "she", "she'd", "she'll", "she's", "should", "so", "some", "such", "than", "that",
"that's", "the", "their", "theirs", "them", "themselves", "then", "there", "there's", "these", "they", "they'd", "they'll",
"they're", "they've", "this", "those", "through", "to", "too", "under", "until", "up", "very", "was", "we", "we'd", "we'll",
"we're", "we've", "were", "what", "what's", "when", "when's", "where", "where's", "which", "while", "who", "who's", "whom",
"why", "why's", "with", "would", "you", "you'd", "you'll", "you're", "you've", "your", "yours", "yourself", "yourselves" ]
train['text'] = train['text'].str.lower().apply(alpha_num).apply(remove_stopwords)
test['text'] = test['text'].str.lower().apply(alpha_num).apply(remove_stopwords)
Training¶
vocab_size = 20000
maxlen = 250
embed_dim = 64
num_heads = 4 # Number of attention heads
padding_type='post'
tokenizer = Tokenizer(num_words=vocab_size)
tokenizer.fit_on_texts(X_train)
word_index = tokenizer.word_index
train_sequences = tokenizer.texts_to_sequences(X_train)
test_sequences = tokenizer.texts_to_sequences(X_test)
trn = keras.preprocessing.sequence.pad_sequences(train_sequences, maxlen=maxlen)
tst = keras.preprocessing.sequence.pad_sequences(test_sequences, maxlen=maxlen)
print(trn.shape, tst.shape)
(54879, 250) (19617, 250)
cv = StratifiedKFold(n_splits=n_fold, shuffle=True, random_state=seed)
def get_model():
ff_dim = 32 # Hidden layer size in feed forward network inside transformer
inputs = layers.Input(shape=(maxlen,))
embedding_layer = TokenAndPositionEmbedding(maxlen, vocab_size, embed_dim)
x = embedding_layer(inputs)
transformer_block = TransformerBlock(embed_dim, num_heads, ff_dim)
x = transformer_block(x)
x = transformer_block(x)
x = layers.GlobalAveragePooling1D()(x)
x = layers.Dropout(0.1)(x)
x = layers.Dense(20, activation="relu")(x)
x = layers.Dropout(0.1)(x)
outputs = layers.Dense(n_class, activation="softmax")(x)
model = keras.Model(inputs=inputs, outputs=outputs)
model.compile(loss='categorical_crossentropy', optimizer=Adam(learning_rate=.001))
return model
p_val = np.zeros((trn.shape[0], n_class))
p_tst = np.zeros((tst.shape[0], n_class))
for i, (i_trn, i_val) in enumerate(cv.split(trn, y), 1):
print(f'training model for CV #{i}')
clf = get_model()
es = EarlyStopping(monitor='val_loss', min_delta=0.001, patience=3,
verbose=1, mode='min', baseline=None, restore_best_weights=True)
clf.fit(trn[i_trn],
to_categorical(y[i_trn]),
validation_data=(trn[i_val], to_categorical(y[i_val])),
epochs=10,
batch_size=128,
callbacks=[es])
p_val[i_val, :] = clf.predict(trn[i_val])
p_tst += clf.predict(tst) / n_fold
clear_session()
gc.collect()
training model for CV #1
Epoch 1/10
343/343 [==============================] - 28s 81ms/step - loss: 1.2887 - val_loss: 0.8706
Epoch 2/10
343/343 [==============================] - 26s 76ms/step - loss: 0.6957 - val_loss: 0.7478
Epoch 3/10
343/343 [==============================] - 26s 75ms/step - loss: 0.4799 - val_loss: 0.8396
Epoch 4/10
343/343 [==============================] - 26s 75ms/step - loss: 0.3655 - val_loss: 0.9340
Epoch 5/10
343/343 [==============================] - ETA: 0s - loss: 0.3008-Restoring model weights from the end of the best epoch.
343/343 [==============================] - 26s 75ms/step - loss: 0.3008 - val_loss: 1.1109
Epoch 00005: early stopping
training model for CV #2
Epoch 1/10
343/343 [==============================] - 26s 76ms/step - loss: 1.2318 - val_loss: 0.8436
Epoch 2/10
343/343 [==============================] - 26s 75ms/step - loss: 0.7035 - val_loss: 0.7588
Epoch 3/10
343/343 [==============================] - 26s 75ms/step - loss: 0.4779 - val_loss: 0.8091
Epoch 4/10
343/343 [==============================] - 26s 75ms/step - loss: 0.3685 - val_loss: 0.8624
Epoch 5/10
343/343 [==============================] - ETA: 0s - loss: 0.3008Restoring model weights from the end of the best epoch.
343/343 [==============================] - 26s 75ms/step - loss: 0.3008 - val_loss: 1.0591
Epoch 00005: early stopping
training model for CV #3
Epoch 1/10
121/343 [=========>....................] - ETA: 15s - loss: 1.5842
---------------------------------------------------------------------------
KeyboardInterrupt Traceback (most recent call last)
<ipython-input-71-18f5cc3689c7> in <module>
8 verbose=1, mode='min', baseline=None, restore_best_weights=True)
9
---> 10 clf.fit(trn[i_trn],
11 to_categorical(y[i_trn]),
12 validation_data=(trn[i_val], to_categorical(y[i_val])),
~/miniconda3/envs/py38/lib/python3.8/site-packages/tensorflow/python/keras/engine/training.py in _method_wrapper(self, *args, **kwargs)
106 def _method_wrapper(self, *args, **kwargs):
107 if not self._in_multi_worker_mode(): # pylint: disable=protected-access
--> 108 return method(self, *args, **kwargs)
109
110 # Running inside `run_distribute_coordinator` already.
~/miniconda3/envs/py38/lib/python3.8/site-packages/tensorflow/python/keras/engine/training.py in fit(self, x, y, batch_size, epochs, verbose, callbacks, validation_split, validation_data, shuffle, class_weight, sample_weight, initial_epoch, steps_per_epoch, validation_steps, validation_batch_size, validation_freq, max_queue_size, workers, use_multiprocessing)
1096 batch_size=batch_size):
1097 callbacks.on_train_batch_begin(step)
-> 1098 tmp_logs = train_function(iterator)
1099 if data_handler.should_sync:
1100 context.async_wait()
~/miniconda3/envs/py38/lib/python3.8/site-packages/tensorflow/python/eager/def_function.py in __call__(self, *args, **kwds)
778 else:
779 compiler = "nonXla"
--> 780 result = self._call(*args, **kwds)
781
782 new_tracing_count = self._get_tracing_count()
~/miniconda3/envs/py38/lib/python3.8/site-packages/tensorflow/python/eager/def_function.py in _call(self, *args, **kwds)
805 # In this case we have created variables on the first call, so we run the
806 # defunned version which is guaranteed to never create variables.
--> 807 return self._stateless_fn(*args, **kwds) # pylint: disable=not-callable
808 elif self._stateful_fn is not None:
809 # Release the lock early so that multiple threads can perform the call
~/miniconda3/envs/py38/lib/python3.8/site-packages/tensorflow/python/eager/function.py in __call__(self, *args, **kwargs)
2827 with self._lock:
2828 graph_function, args, kwargs = self._maybe_define_function(args, kwargs)
-> 2829 return graph_function._filtered_call(args, kwargs) # pylint: disable=protected-access
2830
2831 @property
~/miniconda3/envs/py38/lib/python3.8/site-packages/tensorflow/python/eager/function.py in _filtered_call(self, args, kwargs, cancellation_manager)
1841 `args` and `kwargs`.
1842 """
-> 1843 return self._call_flat(
1844 [t for t in nest.flatten((args, kwargs), expand_composites=True)
1845 if isinstance(t, (ops.Tensor,
~/miniconda3/envs/py38/lib/python3.8/site-packages/tensorflow/python/eager/function.py in _call_flat(self, args, captured_inputs, cancellation_manager)
1921 and executing_eagerly):
1922 # No tape is watching; skip to running the function.
-> 1923 return self._build_call_outputs(self._inference_function.call(
1924 ctx, args, cancellation_manager=cancellation_manager))
1925 forward_backward = self._select_forward_and_backward_functions(
~/miniconda3/envs/py38/lib/python3.8/site-packages/tensorflow/python/eager/function.py in call(self, ctx, args, cancellation_manager)
543 with _InterpolateFunctionError(self):
544 if cancellation_manager is None:
--> 545 outputs = execute.execute(
546 str(self.signature.name),
547 num_outputs=self._num_outputs,
~/miniconda3/envs/py38/lib/python3.8/site-packages/tensorflow/python/eager/execute.py in quick_execute(op_name, num_outputs, inputs, attrs, ctx, name)
57 try:
58 ctx.ensure_initialized()
---> 59 tensors = pywrap_tfe.TFE_Py_Execute(ctx._handle, device_name, op_name,
60 inputs, attrs, num_outputs)
61 except core._NotOkStatusException as e:
KeyboardInterrupt:
print(f'Accuracy (CV): {accuracy_score(y, np.argmax(p_val, axis=1)) * 100:8.4f}%')
print(f'Log Loss (CV): {log_loss(pd.get_dummies(y), p_val):8.4f}')
np.savetxt(p_val_file, p_val, fmt='%.6f', delimiter=',')
np.savetxt(p_tst_file, p_tst, fmt='%.6f', delimiter=',')
시각화¶
print(clf.summary())
Model: "functional_1"
_________________________________________________________________
Layer (type) Output Shape Param #
=================================================================
input_1 (InputLayer) [(None, 500)] 0
_________________________________________________________________
token_and_position_embedding (None, 500, 64) 1312000
_________________________________________________________________
transformer_block (Transform (None, 500, 64) 21088
_________________________________________________________________
global_average_pooling1d (Gl (None, 64) 0
_________________________________________________________________
dropout_2 (Dropout) (None, 64) 0
_________________________________________________________________
dense_6 (Dense) (None, 20) 1300
_________________________________________________________________
dropout_3 (Dropout) (None, 20) 0
_________________________________________________________________
dense_7 (Dense) (None, 5) 105
=================================================================
Total params: 1,334,493
Trainable params: 1,334,493
Non-trainable params: 0
_________________________________________________________________
None
plot_model(clf)
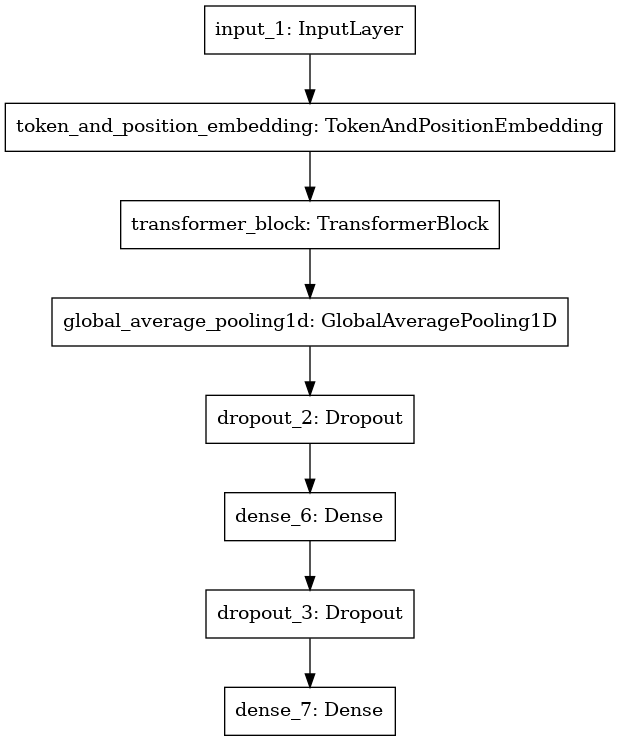
제출 파일 생성¶
sub = pd.read_csv(sample_file, index_col=0)
print(sub.shape)
sub.head()
(19617, 5)
0 | 1 | 2 | 3 | 4 | |
---|---|---|---|---|---|
index | |||||
0 | 0 | 0 | 0 | 0 | 0 |
1 | 0 | 0 | 0 | 0 | 0 |
2 | 0 | 0 | 0 | 0 | 0 |
3 | 0 | 0 | 0 | 0 | 0 |
4 | 0 | 0 | 0 | 0 | 0 |
sub[sub.columns] = p_tst
sub.head()
0 | 1 | 2 | 3 | 4 | |
---|---|---|---|---|---|
index | |||||
0 | 0.0474 | 0.3432 | 0.4971 | 0.0956 | 0.0166 |
1 | 0.0958 | 0.6825 | 0.0563 | 0.0509 | 0.1145 |
2 | 0.9958 | 0.0009 | 0.0005 | 0.0002 | 0.0026 |
3 | 0.0559 | 0.0050 | 0.7425 | 0.0032 | 0.1934 |
4 | 0.5421 | 0.0157 | 0.0246 | 0.4000 | 0.0176 |
sub.to_csv(sub_file)